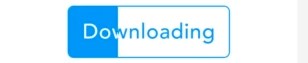
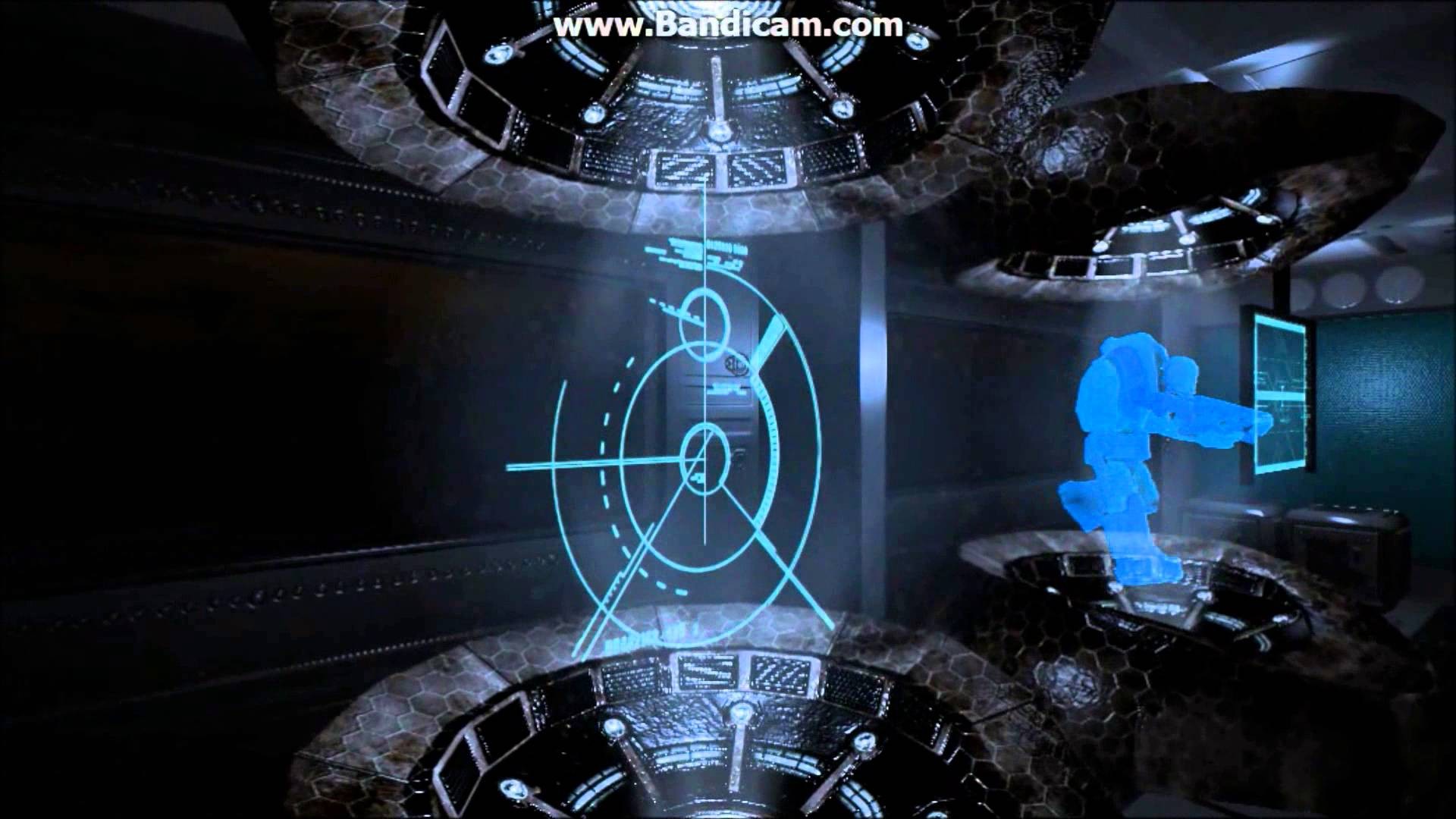
In this post, we will tackle the problem of medical image segmentation, focused on magnetic resonance images, which is one of the most popular tasks, because it is the task with the most well-structured datasets that someone can get access to. This work serves as an intersection of these two worlds: Deep neural networks and medical imaging. That’s why it is estimated that AI and deep learning in medical imaging will create a brand new market of more than a billion dollars by 2023. Consequently, it is obvious that the first three causes of human deaths are related to medical imaging. Medical errors are the third-leading cause of death, after heart disease and cancer in the Another reason that this field attracts a lot of attention is its direct impact on human lives. Nevertheless, the application-specific data are still not available it is clear that AI will hugely impact the evolution of medicine through medical imaging.Īs we will see a medical image is often three or four-dimensional. A lot of researches have already attempted to automatically detect COVID-19 through deep networks from 3D CT scans. Recently, Imperial College of London launched a course on COVID-19.

Even though medical imaging data are not so easy to obtain, DNN’s seem to be an ideal candidate to model such complex and high dimensional data. In the generalized task of image recognition, which includes problems such as object detection, image classification, and segmentation, activity recognition, optical flow and pose estimation, we can easily claim that DNN (Deep Neural Networks) have achieved superior performance.Īlong with this rise in computer vision, there has been a lot of interest in the application in the field of medical The rise of deep networks in the field of computer vision provided state-of-the-art solutions in problems that classical image processing techniques performed poorly. Introduction to Deep Learning & Neural Networks with Pytorch Deep learning and medical imaging
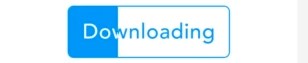